1. Introduction
Speech intelligibility in general refers to the ability of spoken language to be understood by listeners, and it is an important consideration in language assessment, as it is often used as a criterion for evaluating pronunciation. The idea that a comfortably intelligible pronunciation and robust communicative skills are sufficient for language learners has gained support in the literature (Abercrombie, 1949; Brown, 1989; Derwing & Munro, 2015; Munro, 2010). For example, Abercrombie (1949) discussed whether retaining native-like pronunciation is necessary to language learners and argued that most language learners only require “a comfortably intelligible pronunciation” which he defined as “a pronunciation which can be understood with little or no conscious effort on the part of the listener (p. 37)”. In addition, both research and practice turned their attention to intelligibility referring to empirical evidence suggesting only few adult learners achieve native-like pronunciation in the L2 (Flege et al., 1995).
However, establishing intelligibility as a pronunciation standard remains challenging, a longstanding issue in linguistic studies (Brown, 1989; Jenkins, 2000; Lado, 1961; Levis, 2010). For example, intelligibility and accuracy in speech often intersect; higher pronunciation accuracy may enhance intelligibility, influenced by clear articulation, speech context, and listener familiarity with the spoken language. Thus, improving intelligibility, a pivotal element in pronunciation assessment, necessitates a diverse approach and effective pronunciation assessment is key in language education, boosting learner confidence, motivation, and learning outcomes.
Nevertheless, the complexities of pronunciation assessment, especially in rigid-curriculum settings with large student numbers and limited time, often hinder detailed assessments requiring individualized feedback. In addition, the inherent subjectivity of human evaluators, influenced by factors such as their first language (L1), introduces biases into the assessment process (Carey et al., 2011; Winke et al., 2013). Furthermore, not all teachers, particularly non-native English speakers, may have received adequate training or possess the necessary expertise, affecting the accuracy of assessments. This makes it challenging to provide meaningful feedback on English learners’ speech, especially about complex or nuanced aspects of pronunciation and communicative skills. To address these challenges, educators might incorporate pronunciation assessment into their curriculum using technologies like speech recognition software for efficient assessment and immediate feedback.
Researchers have explored potential of automated speech recognition (ASR) technology in English pronunciation assessment for its promise of objectivity and consistency (Baralt et al., 2011; Derwing et al., 2000; Hincks, 2003; Yang, 2020). ASR technology, a software that uses consistent algorithms to analyze and recognize spoken language, has been widely used in various applications, including voice assistants, language translation, and speech-to-text (STT) transcription. The use of ASR technology in language education has been ongoing since the early 21st century (Park, 2017; Park et al., 2016). However, due to the lack of notable technological advancements at that time, it is difficult to compare it with recent research.
In one of the recent studies, Yang (2020) assessed the pronunciation of Korean English learners using Speechnotes application and native English raters. The study found an average correct word recognition rate of 77.7%, suggesting intermediate or higher pronunciation skills. The correlation between correctly recognized content words and raters’ scores was moderate, while it was negligible for function words. The study concluded that while Speechnotes can partially diagnose pronunciation issues, further research is needed to align it with human raters. Similarly, Hong & Nam (2021) evaluated the reliability of the commercial ASR-based pronunciation system, SpeechPro, by comparing it with human raters. They found that the machine-human score agreement was similar to human-human agreement across all metrics, validating the score reliability of SpeechPro for comparison with other systems.
In a study exploring the educational effects of using ASR tools, Spring & Tabuchi (2022) investigated the effectiveness of using ASR tool in an online English as a foreign language course for Japanese students to improve their pronunciation. They reported that the ASR tool was found to be particularly useful for students with less than 95% accuracy on the pretests. This could imply that the ASR tool may be particularly effective in helping students who are struggling more with their pronunciation, as indicated by their pretest scores. In addition, students who participated in the study reported that it was most helpful for practicing consonant and vowel sounds.
As ASR technology rapidly evolves, technology-based pronunciation assessment is becoming more prominent. Given recent advancements in speech synthesis and recognition technology with AI, further research is needed to explore the value of these digital tools. In addition, despite the ‘black box’ nature of ASR systems often perplexing humanities researchers, ASR technology is instrumental in language research due to its capability to transcribe large volumes of spoken language data efficiently. Its evolving precision enhances reliability, and despite the complex, opaque algorithm, the significant advantages it offers to linguistic analysis underscore its substantial value. Building on this, ASR tools possess considerable potential in pronunciation assessment, a crucial facet of language research.
Nevertheless, further investigation is necessary to ascertain their effective utilization and reliability, thereby ensuring these resources contribute optimally to the study and understanding of language. In particular, pronunciation assessment should extend beyond a mere summative tool; it should act as a formative guide, diagnosing and providing feedback to learners, and tracking speaking progress. However, the necessary time and educator effort can be overwhelming. Therefore, the exploration of efficient, referenceable pronunciation assessment methods is pivotal in enhancing speaking education.
To address the challenges of time and educator effort as well as the needs for learner feedback, this study explores the application of ASR technology, Whisper (OpenAI, 2023), in assessing English pronunciation. This open-source tool, accessed through the Python coding platform, allows for a systematic evaluation of learner speech processing. It enables a direct comparison of ASR with human raters, offering a new perspective on pronunciation assessment. Finally, we expect that the non-commercial nature of Whisper makes it a practical resource for all users, from educators to students, without necessitating extensive ASR expertise.
The article is organized as follows: Section 2 outlines the research methodology, including data collection, ASR digital tools, and evaluation criteria. Section 3 reports the results, compares the ASR’s Word Error Rate (WER) scores with those of human evaluators, and discusses the effectiveness and applicability of digital tools in education. Section 4 concludes by summarizing the findings, acknowledging limitations, and suggesting directions for future research.
2. Methodology
This study employs a methodology that uses speech data from English learners and synthesized speech generated by the freely available Google Text-to-Speech engine (gTTS, ver. 2.3.2), which allows users to generate both typical English and Korean-accented English. We selected synthesized speech, with its capability to generate typical Korean-accented English pronunciation, to assess the degree of speech recognition through ASR. Both the synthesized and learners’ speech were evaluated using Whisper (ver. 1.1.10), an ASR tool, along with human raters in the current English education system. The implementation of digital tools was employed with Python programming language (Van Rossum & Drake, 2009).
Speech samples were collected from college-level pronunciation classes (N=30 freshmen, 15 females and 15 males) and a native English speaker, a female college lecturer in her late 20s from the southern USA. Students self-recorded their speech for a diagnostic test at the start of the course and submitted recordings of the same material for a formative assessment at the end.1 For the reading material, we utilized a set of 19 test sentences, amounting to 331 words, recommended in a pronunciation textbook used for the course (Dale & Poms, 2005). We also incorporated the Rainbow passage (Fairbanks, 1960), consisting of 19 sentences as shown to generate our speech synthesis to test ASR.
The Rainbow passage:
“(S1) When the sunlight strikes raindrops in the air, they act as a prism and form a rainbow. (S2) The rainbow is a division of [...] (S19) This is a very common type of bow, one showing mainly red and yellow, with little or no green or blue.” (1)
Another source of speech data is obtained through speech synthesis (gTTS). The purpose of using synthesized speech is to set reference points between native-like speech and heavily accented speech. By referring to these types of speech, we can estimate the potential baseline of ASR results. Thus, synthesized speech is used to guide our ASR tool for a practical purpose.
Perception experiments are conducted to compare pronunciation assessment by human raters and ASR performances. The speech recordings have two conditions: before the course (PRE) and after the course (POST). Considering the listening load of the perception experiment by human raters, only one of the 19 test sentences is chosen for the perception experiment: “The programs about detectives and hospitals are my favorites.”2 A total of 180 stimuli were used in each of the two perception experiments: 30 (subjects)×2 (PRE/POST conditions)×3 (randomized repetition). The experiment was constructed using Praat (Boersma & Weenink, 2023) MFC. In Exp1, raters were asked to assess proficiency and comprehensibility for the 180 stimuli while listening to each stimulus. There were breaks after every 60 stimuli. In the following Exp2, the same raters were evaluated the same stimuli (randomized) in terms of accuracy and intelligibility. All four aspects of pronunciation were asked on a scale of 7. Thus, for each rater, they listened 180 stimuli twice to assess the four concepts.
Four human raters, currently teaching English at high schools or universities, participated in this pronunciation assessment experiment. These raters had taken a pronunciation assessment course as a part of their graduate course work, during which they spent eight weeks discussing concepts like intelligibility, accuracy, comprehensibility, and other factors necessary for pronunciation assessment. As outlined in Derwing & Munro (2015).
The outcomes of the perception experiment will be compared with ASR results to evaluate the practical applicability of ASR in pronunciation assessment, as well as the immediate potential of digital tools for assistance in educational settings.
gTTS is the speech synthesize engine that converts text into spoken data. It offers an Application Programming Interface (API) that allows users to utilize the tool in the synthesis process using Python coding. Using the API with Python coding enhances researchers’ efficiency and flexibility, facilitating the integration of functionalities and services, saving time and effort while enabling customized workflows for specific research needs.
This paper leverages this accessibility to generate artificial speech, establishing recognition reference points for using ASR tools. English speech (language=“en-us”) synthesized from the gTTS closely resembles native speech to the human ear and is expected to be accurately recognized by ASR. Conversely, Korean-accented speech exhibits significant phonological transfer, as predicted by contrastive analysis or error analysis (Archibald, 1998; Lado, 1957). For instance, in the context of a typical Seoul Korean accent and with the language setting in gTTS set to Korean (language=“ko”), English words like ‘meat’ and ‘speech’ are generated as [mi.thɯ] and [sɯ.phi.tshi], respectively, with vowel insertions in the consonant clusters /sp/ and the final consonants /s/ and /tʃ/ in the given words. For English words containing phonemes absent in Korean, substitutions of other consonants are used, such as /pa.i.bɯ/ for ‘five’. This speech bears Korean-specific rules for naturalness and intelligibility. With the accented speech, we can establish a reference scale for the recognition of typical Korean accented speech obtained from gTTS and calculate WER values based on the ASR performance.
Whisper, a STT engine or ASR engine, is a digital tool that converts spoken language into written text. It employs algorithms and models to analyze and transcribe audio input.3 This tool can also be utilized in the recognition process with Python coding, as it an offers API similar to gTTS.
Note that our intention in using digital tools is not to assess technical performance or functionality. Instead, we aim to explore the practical value of these tools for experts with limited familiarity with technology development. By focusing on the usefulness of these digital tools to non-technical experts, we aim to identify how they can enhance productivity or provide valuable insights in the educational domain.
The ASR performance was evaluated by comparing its accuracy in transcribing native and Korean-accented English speech samples. This aimed to assess its ability to handle different accents and dialects. Performance was measured using the WER as shown in (2), a percentage reflecting the minimum number of edits (insertions, deletions, or substitutions) needed to match the ASR output to the original speech, similar to the Levenshtein distance metric used in tasks like spell-checking and string similarity determination (Levenshtein, 1966; Schulz & Mihov, 2022):
Each error type signifies a different system mistake: substitutions for incorrect words, deletions for missing words, and insertions for extra words. A lower WER indicates better performance, with 0 representing perfect transcription and 1 complete misrecognition, potentially exceeding 1 with more insertions. Note that the problem of determining the source of a mispronounced word, the user, or the recognition system, is a significant challenge in evaluating a system’s performance, regardless of the metric used. This is particularly relevant in systems designed to handle non-native speakers or those with strong regional accents.
3. Results and Discussions
We used the Whisper tool for speech recognition on three types of speech: synthesized English (SynE), synthesized Korean accented speech (SynK), and a recording from a native English speaker (HumE). Table 1 displays the results, providing a baseline for subsequent comparisons with learners’ speech data.
Data | Audio from | WER | Runtime (sec.) |
---|---|---|---|
SynE | Synthesized | 0.000 | 2.186 |
SynK | Synthesized | 1.333 | 2.478 |
HumE | Human | 0.000 | 2.048 |
For the sentence used in the perception experiment, the Whisper tool perfectly recognized the HumE and SynE (WER=0.000) but made multiple errors with the SynK (WER>1).4 For instance, the sentence, “The programs about detectives and hospitals are my favorites” produced in synK, was recognized as “Torre programs are about detectives and who spit a say I might pay for it, sir.” This result is far from the original sentence, illustrating how the tool, like a human listener, tries to make sense of what it identifies. In this sense, the result is not based on the concept of accuracy, but rather closer to the concept of intelligibility or comprehensibility.
With these WER scores as reference points, we can now compare the test sentences (the rainbow passage) to expand our reference points. The result of the ASR recognition is shown in Table 2, where HumE is a recording from one native speaker of English obtained for a comparison:
Data | Audio from | WER (Mean) | WER (SD) |
---|---|---|---|
SynE | Synthesized | 0.0 (0.017) | 0.032 |
SynK | Synthesized | 0.7 (0.744) | 0.314 |
HumE | Human | 0.0 (0.024) | 0.036 |
In the table above, we observe that both synthesized and human speech data are recognized almost perfectly, with WER values close to 0. On the other hand, the synthesize Korean-accented English data shows 0.7 (ranged from 0.1–1.1) in WER, which is obviously closer to 1 with the sign of multiple errors. We will now examine the stimuli used in the perception test. They were obtained from 30 learners under two conditions (PRE vs. POST), resulting in a total of 60 utterances.
The combined WER result from ASR process did not show any statistically significant difference when examined all subjects, and this may not be surprising. Pronunciation instruction has been found to improve L2 accents in some learners, but not all (Kissling, 2013). Furthermore, while there are noticeable differences in pronunciation features between high- and low-proficiency English learners, these differences are not always evident between adjacent proficiency levels. This implies that the enhancement of pronunciation skills does not necessarily correspond directly with assessed proficiency levels. In addition, our data may exhibit a potential ceiling effect (approaching WER=0), indicating that some learners’ recordings were already of sufficiently high quality for automated recognition. As discussed earlier, the ASR tool could be beneficial to students with lower proficiency (Spring & Tabuchi, 2022).
To investigate potential pronunciation learning effects in more detail, we applied a criterion to select the data, excluding learners whose pre-test WER was below 0.111, indicating an error(s) in at most one word out of the 9-word sentence. In Figure 1 below, we observed that post-test recordings were better recognized in the ASR process, showing lower WER values for 18 subjects. We also find a higher WER score in the PRE condition compared to the POST, indicating potentially poorer performance before taking a course.
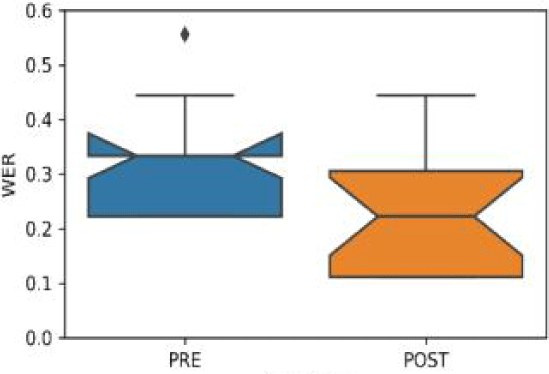
The mixed linear model regression results reveal a positive coefficient (0.09, se=0.035) for the condition, indicating an improvement in the ASR performance from the PRE to the POST condition. The z-score of 2.849 (p=0.004) confirms the statistical significance of this effect. In addition, the coefficient for gender (male) is –0.55 (se=0.059) and the z-score is –0.927, indicating no significant gender effect (p=0.354). These findings highlight a significant difference in WER between the PRE and POST conditions.
Individual changes in WER are shown in Figure 2. In this figure, we see that most learners had lower (less errors) or the same WER values in the POST condition with some exceptions. This does not directly indicate that the learners improved their pronunciation according to human. Rather, it means that automated recognition is better for the training, and the speech in the POST condition could fit better to the native model ASR.
Once again, it is not saying learners should perform like native speakers of English. This improvement can assist learners in finding directions for self-learning.
This section examines the perception results conducted by four human raters. The experiment was divided into two sessions: Experiment 1 asks proficiency levels and comprehensibility, and Experiment 2 asks accuracy and intelligibility. For the proficiency level, 1 is marked as ‘Beginner’, 4 as ‘Intermediate’, and 7 as ‘Advanced’. Each rater listened to a total of 180 stimuli in each session and rated individual factors on a scale of 1 to 7.
The mixed linear model regression analysis of proficiency assessment revealed no significant effect for the condition (PRE vs. POST) or repetitions. However, it shows significant effects for gender as the coefficient for gender was –0.769 (se=0.236, p=0.001), indicating a lower average level of the dependent variable for males compared to females. Similar results are found for accuracy, intelligibility, and comprehensibility, indicating a gender difference in the given data with significantly lower for males on the four measures. Note that this should not be interpreted as a direct gender effect, as the learner samples were neither random nor controlled.
To examine further assessment details of learner speech and human raters, we focus on the sub-data by removing learners whose speech was readily good before the training, potentially making a ceiling effect. The threshold for selection is set to points lower than 6 (1–7 scale) in the sub-data. For these analyses, the number of items selected differs among proficiency, comprehensibility, accuracy, and intelligibility data sets (Nprof=672, Ncomp=600, Nacc=648, Nintel=600). The fixed effect is set to the four measures and random effects are subjects, repetitions, and raters.
Proficiency: The mixed linear model regression analysis of 672 observations showed a significant baseline value for proficiency (intercept coefficient of 4.885, p<0.001). The PRE condition and being male were associated with significantly lower values (coefficients= –0.199, p=0.001 and –0.687, p=0.003, respectively). As shown in Figure 3 below, HR2 showed significantly lower proficiency, while HR3 showed significantly higher proficiency.
There was a significantly lower value of proficiency associated with HR2 (coefficient= –0.821, p<0.001) while a significantly higher value with HR3 (coefficient=1.280, p<0.001). Rater differences will be discussed in 3.2.2 with correlation matrix. Note that the gender effect should be disregarded in this analysis as the sample was not randomly selected, and the learners’ proficiency levels were not controlled or standardized (coefficient= –0.687, p=0.003).
Comprehensibility: There are 672 observations in the sub-data of proficiency (intercept=4.885). Similar results are found for comprehensibility rating. We find significant effects for conditions (PRE/POST) and gender. Female learners had higher ratings even in the PRE condition (conditions coefficient= –0.213, se=0.082). A z-score of −2,589 (p=0.01) indicates a significant difference in comprehensibility ratings between conditions. For the raters, the similar tendency is found between HR2 (lower ratings) and HR3 (higher ratings).
Accuracy: Analysis of 648 items showed an average accuracy value of 4.823. Except the gender effect, no significant difference was found between conditions (coefficient= –0.065, se=0.082, z= –0.794, p=0.427). It appears that there might be a correlation between the repetitions (R2 and R3) and an increase in the dependent variable’s values. However, this observation is only of marginal significance.
Intelligibility: Analysis of 600 items showed an average intelligibility rating of 4.842. No significant difference was found between PRE and POST conditions (coefficient= –0.097, p=0.217). except the gender effect. Among the repetitions, R3 was associated with a higher value on average (p=0.039). Among the raters, HR2 is associated with lower values while HR3 with higher values (p<0.001).
When human raters are involved in data measurement, it is common to report inter-rater reliability using a statistical measure, such as Cohen’s kappa. This measure assesses the level of agreement between two raters or observers when coding or categorizing data. The kappa values among the raters in this study all vary, ranging from 0.1 to 0.4, depending on the target measure. However, this does not mean that the rating data is unreliable.
The raters in this study are highly proficient in English, have necessary phonetic and phonological knowledge. They are all experienced language teachers in public schools for many years. In practice, no teacher will have co-raters in assessing their own students. Thus, the assessment process in this study did not involve using rubrics, letting potential factors other than segmental, prosodic, and rhythmic elements involving in this pronunciation assessment. The raters also shared definitions in the literature, case studies discussed in Derwing & Munro (2015), as well as their own experiences and examples. They are aware that individual speaking habits, speaking styles, speech rate, pauses, and voice quality can still impact ratings in terms of accuracy, proficiency, intelligibility, and comprehensibility.
We will now analyze the strategies and influences of each rater in the pronunciation assessment process. Correlation analyses were conducted among the rating results (proficiency, accuracy, comprehensibility, and intelligibility) to determine the strength and direction of the relationship between these variables. The results indicate that as the WER decreases (improved performance), proficiency, accuracy, and intelligibility tend to increase, as depicted in Figure 4.
More specifically, WER and comprehensibility has a strong negative correlation (r= –0.57, p<0.001), a moderate correlation with accuracy (r= –0.52, p < 0.001), intelligibility (r= –0.54, p<0.001), and proficiency (r=−0.52, p<0.001). The all four measures are correlated with WER measure produced by ASR, but they are all relatively similar across the measures. Human raters, on the other hand, demonstrate strong correlations among the measures, as depicted in Figure 5.
Proficiency and intelligibility have the strongest correlation across all raters, with individual variations noted. For example, HR2 and HR4, show the highest correlations between proficiency- comprehensibility and comprehensibility-intelligibility. These patterns contrast with the WER result from the ASR, suggesting that automated systems may not align with individual rater biases, possibly accounting for the low Cohen’s kappa among the raters. This interpretation, while not definitive, calls for further investigation.
Traditional language assessment methods, which heavily rely on human raters for pronunciation teaching and evaluation, can be time-consuming and inefficient. Empirical evidence shows that human raters typically take between 3.2 to 6 seconds to assess a single sentence, excluding listening time. In the experimental design, the audio was configured to play only once.
The ASR engine (‘Whisper’ setting to ‘base.en’ model) used in this paper took 33 seconds to process 19 different speech files, which are Korean-accented and have more words in each utterance. Processing one sentence took approximately 1.7 seconds or less. It should be noted that the reaction time is intended to estimate the approximate time it took for the evaluator to assess the utterance and may not be reaction time in the strictest sense (Figure 6).
The substantial time savings observed with the use of digital tools has far-reaching implications for many practical purposes. By automating the laborious process of pronunciation evaluation, teachers, for example, can allocate their valuable time more effectively to other critical aspects of language teaching. Thus, these tools not only enhance efficiency but also augment the overall quality of instruction.
4. Conclusion
The findings from the current study reveal meaningful insights into the efficacy and efficiency of ASR systems vis-à-vis human raters in the context of language assessment. Our examination began with an exploration of the performance of ASR on the sample data, and we observed the capabilities of the ASR system in handling varied speech inputs (native and accented speech) and making proficient evaluations.
Our examination of learner speech, specifically targeting those with ratings under 6 on a scale of 1–7, unveiled noteworthy results in proficiency, comprehensibility, accuracy, and intelligibility. Proficiency and comprehensibility varied significantly between conditions, unlike accuracy and intelligibility. Individual rater analysis showed variations and a contrasting pattern with the WER from ASR, suggesting potential rater biases. Acknowledging the constraint of limited test materials in the perception experiment with human raters, this analysis found learners’ higher ratings from human raters and better recognition from ASR post-pronunciation training, offering valuable insights into their performance.
Demonstrating improvement in speaking or pronunciation of learners poses challenges, particularly considering the prevailing notion that explicit pronunciation teaching offers limited value and effectiveness (Derwing et al., 2002; Purcell & Suter, 1980). Bridging the gap between theoretical research and practical teaching has been difficult due to the lack of clear guidelines. To address this, our study focused on a group of learners requiring improvement, identified by rating scores below 6, indicating a need for progress towards advanced or native-like levels. We conducted a comparative analysis between human raters and the ASR system, considering proficiency, accuracy, intelligibility, and comprehensibility.
The current study highlights ASR technology’s potential in streamlining traditional language assessment. Human raters spend 3.2 to 6 seconds per sentence, excluding listening time, raising efficiency and scalability concerns. In contrast, ‘Whisper’ ASR engine processes a longer sentence in about 1.7 seconds with a base model, emphasizing its efficiency. Accordingly, ASR systems and digital tools can enhance language education, improving task efficiency and promoting unbiased instruction.
Using ASR for pronunciation assessment poses challenges. The complexity of ASR development often prevents language researchers and educators from fully grasping its principles and variables, especially when its methodology diverges from established techniques and frameworks. Additionally, using these models requires coding skills and significant training. While commercial digital tools are user-friendly, they offer limited flexibility. As voice recognition technology evolves and becomes more intertwined with programming and API usage, improving digital literacy among researchers and educators is crucial. To make voice recognition a useful tool for language research and learning, continuous validation of its validity and practicality by developers, researchers, and educators can be essential.
In conclusion, ASR tools hold potential for efficient pronunciation assessment, offering insights into technology-assisted evaluation. While they cannot fully replace human evaluators due to their technical complexity, they represent a significant step forward in language teaching. Future research should utilize these tools to improve pronunciation assessment. As technology advances, further exploration is essential to fully comprehend their role in language teaching and assessment, potentially revolutionizing this field.